News
Development and Validation of a Deep Learning Model for Detection of Allergic Reactions Using Safety Event Reports Across Hospitals
Nov 16, 2020
By applying a deep learning algorithm to over 299,028 safety reports filed at Brigham and Women’s Hospital and Massachusetts General Hospital, researchers were able to identify patient allergic reactions in electronic medical records with greater frequency and accuracy than a keyword search approach. The algorithm was able to identify 24.2% more cases of confirmed allergic reactions and reduced the need for manual review by 63.8%.
The findings suggest that similar deep learning models may improve the accuracy and efficiency in identifying allergic reactions in hospitals, which could greatly benefit surveillance and guidance for medical errors and system improvement.
Citation for the Full-text Article
Yang J, Wang L, Phadke NA, et al. Development and validation of a deep learning model for detection of allergic reactions using safety event reports across hospitals. JAMA Network Open. 2020;3(11):e2022836. DOI:10.1001/jamanetworkopen.2020.22836
Latest News from CRICO
Effects of Poor Communication in Healthcare
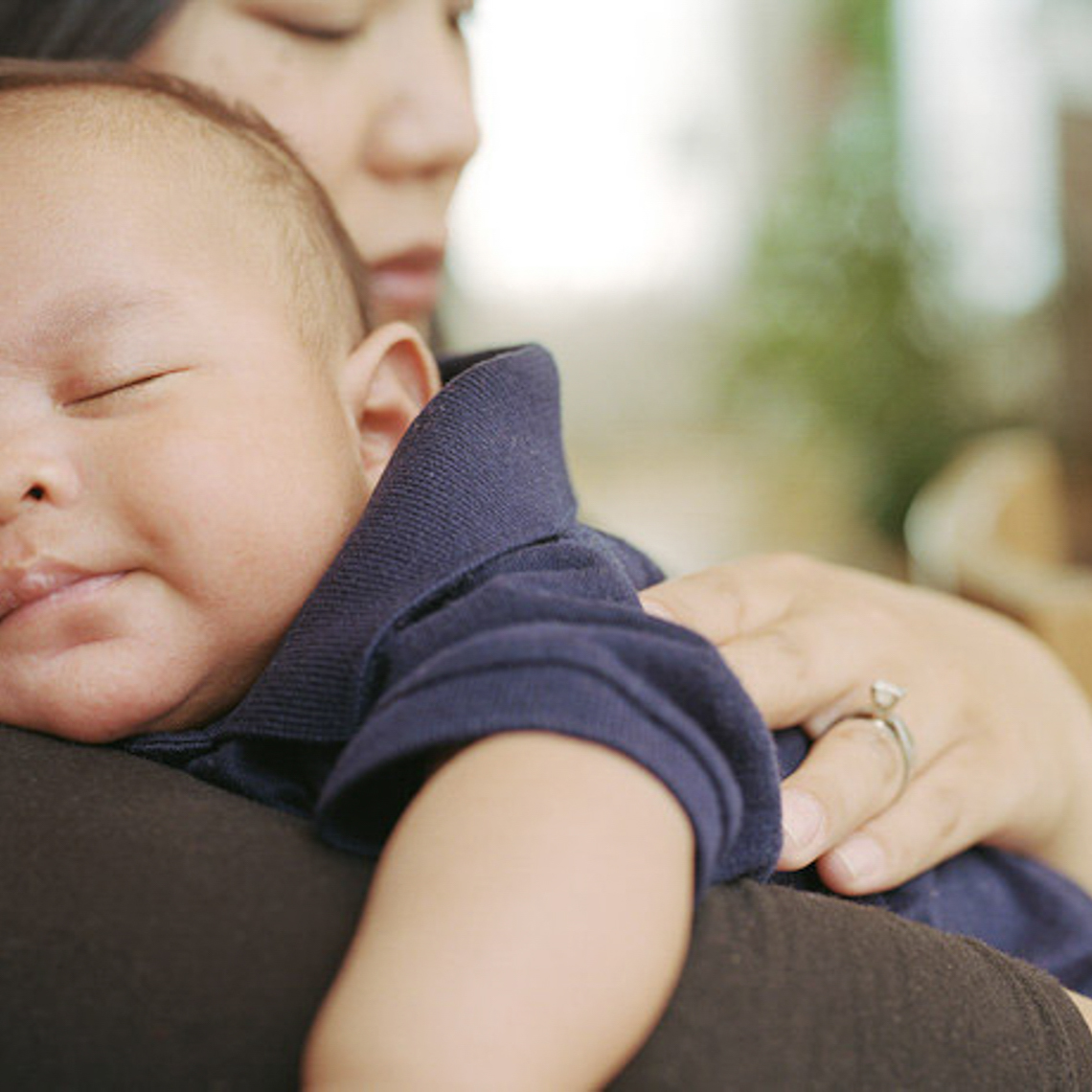